5 Things to Know Before Starting a Member Data Project
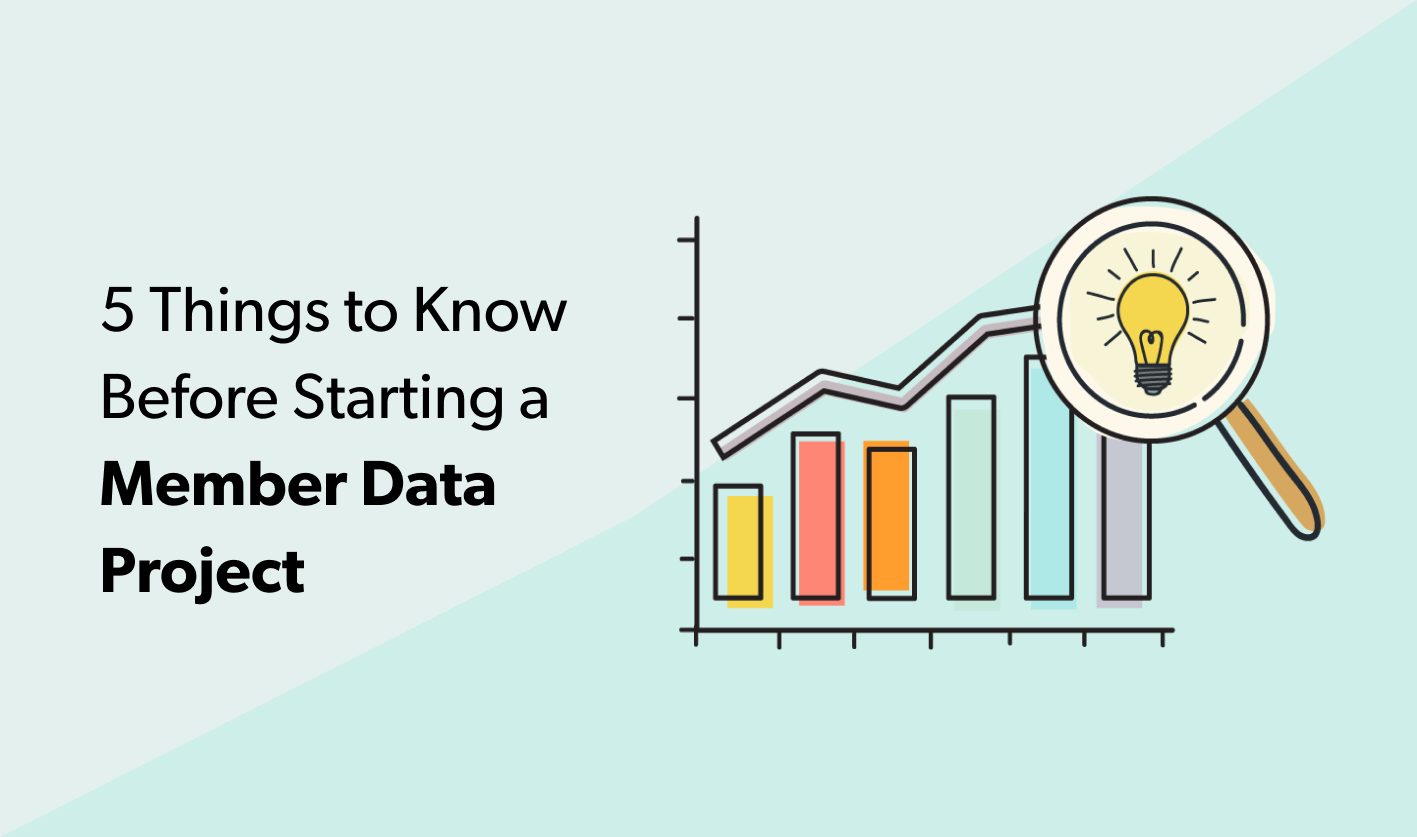
Recently, Higher Logic teamed up with Wicket and CSAE for a popular webinar series, Let’s Talk About Member Data. The series covered the basics of member data and how to turn that data into actionable information. Three webinars weren’t nearly enough to cover all things data, but it was a great start.
You can view the three webinars individually here:
- Session 1: Member Data 101
- Session 2: Exploring Your Data
- Session 3: Turning Insight Into Action
I’ve also put together a list of the five most important takeaways from the series:
- Data requires maintenance.
- Data should be validated.
- Know what you want to do with the data.
- Benchmark against yourself.
- Data is nothing without context.
Let’s dive in.
1. Data requires maintenance.
Anytime you collect data, you need to make sure you’re using the right tools to keep it secure. The more tools in your tech stack, the more opportunities to lose track of your data. And the more systems you have, the more likely you are to lose track of things like opt-outs, which are critical to complying with laws and regulations.
Fix it: Invest in tools that integrate. For example, Higher Logic is an online member community and communications platform that integrates with many systems, including Wicket’s member data platform. This makes collecting, managing, and using all of your member data in one place much easier.
2. Data should be validated.
The more mission-critical the decision you or your association are trying to make, the more important it is to validate your data. You have to know exactly what kind of data you’re using so that when you present the results, everyone is on the same page.
It’s a time-consuming step, but it’s actually the most important. You never want someone to say, “Wait, how did we get here?”
It’s amazing how much time can get absorbed into the details like logic and thought process. But that’s what makes the data accurate. When you’re drilling down on things that have a high level of value, you need additional visibility on those decimal points.
– Joshua Slyman, Sr. Consultant at Higher Logic
Here are three steps to validating your data:
Step #1: Identify Data Types
First, you need to know exactly what data you’re working with.
If you’re like most associations, you probably have older databases or a mix of places where you store your data. Every time you do a data project at your association, you need to go through that information to understand which data is important, how it was measured, and whether or not that’s different across other data points.
Step #2: Determine Data Quality
Once you know what type of data is available, you can begin to assess the quality of the data.
One example many associations run into is membership information and renewals. Do you have data you can actually use, or is it a jumble of typed-out cancellation reasons that will take a while to dig through?
Evaluate your data based on these criteria:
- Completeness: How much data do you have? Is it complete for every field you’re looking at?
- Timeliness: How recent is this data? Have you made major changes to your association since the data was collected? How are you collecting the data in the first place?
- Validity: How does a member interact with this data? What options exist, or is it free-form?
- Consistency: How often is this data point used and filled out? How relevant is this data point to the overall question?
- Integrity: How can you double-check to make sure this data is accurate?
Step #3: Use Aggregation Methods
Once you know what type of data you have and what quality you’re working with, it’s time for the final step – determining the central tendency of your data.
Measuring the central tendency of your data is a fancy way of saying “Where is most of the data in your data set?” The main metrics to look at are:
- Mean: Synonym for average. You can calculate the mean by taking the sum of all of the values in the data set and dividing by the number of the data set.
- Median: Refers to the middle. If you were to line up every single value from left to right, the median would be the one directly in the middle.
- Mode: Most frequently occurring value in the data set.
- Frequency Distribution: This shows you how to spread out your data set is, often through a visualization called a histogram.
- Measurement of Spread: Gives you an idea of the range of values you’re working with, including the minimum, maximum, and any outliers. Often visualized through box-and-whisker-plots.
Aggregation methods help you assess the quality of your data today and how long it will be useful.
3. Know what you want to do with the data.
Whatever question you ask needs to have an action behind it. As soon as you have the right question, what are you going to do with the answer? How often do you need to revisit it and why? Ask yourself these questions to define the actions you need to take:
Refining Question & Action
- How frequently do I need to know this?
- Is there a particular format I need to view the data in?
- Who else needs to see the data?
- Do I need to interact with the data to meet the need, such as drilling down into more detail? Is there slicing and dicing I need to do?
- Are there extra details I would need to move forward with the action?
- Is the action informing a decision, prompting a task, or driving change?
4. Benchmark against yourself.
Ultimately, every association is different. It’s great to hear about benchmarks and best practices for context, but the best place to find answers is within your own data.
You need to benchmark against yourself. What’s performing well compared to past data? What’s not? What do you need to change to see improvement in your own data?
When you build your own structure, you give yourself so much more flexibility to change your activities and actions on the fly, since it’s your initiatives that are going to move the needle on those benchmarks.
When you’re ready to dive into validating your data, follow these steps:
- Think about the level of accuracy you’ll need
- Create a plan to direct your validation efforts
- Work validation time into planning
- Document the results of your validation
- Be prepared to modify your plan
- Don’t reinvent the wheel
5. Data is nothing without context.
Why benchmark against yourself? Because data is nothing without context, and you only have the context for your members. Did your member recruitment numbers take a nosedive in April because it was the month after your annual conference and lots of people join for the annual? Or was it a normal month, and you need to look elsewhere for the explanation?
Context is everything when it comes to data. And the context, like your membership, is going to be unique to your organization.
Now that you have these takeaways in hand, you’re ready to get that member data project rolling. If you have questions or want to learn more, listen in to the full webinar series: Let’s Talk About Member Data.